AIOps: Launching with Purpose!
Chris Cosentino, President of Contender Solutions
What is AIOps?
By definition, AIOps is artificial intelligence for IT operations. More tactically speaking, it is the use of machine learning and machine reasoning techniques to improve efficiency, performance, and scalability in IT operations. AIOps requires explaining the application of artificial intelligence and the result to humans so we can understand and trust the outcome.
AIOps is here!
According to a study by Future Marketing Insights, the AIOps platform market is expected to reach $80.2 Billion by 2032, growing at a CAGR of 25.4% from 2022 to 2032.
These facts are intriguing as the market is so vast, yet most companies are as confused today as they were years ago on how to successfully adopt AI into their operational capabilities.
With this growing market, buyers are motivated to invest in technology, thinking that the product provides the “WAM BAM” solution, when ultimately the success lies within the data quality! A Deloitte survey recently found that AI adopters were challenged by lack of transparency along with worries regarding poor decision making based on AI recommendations.
Operational Impact of a maturing AIOps Program
In lower levels of automation maturity, event sources monitoring critical applications and supporting infrastructure are integrated to a system of engagement. When an event arises, the monitoring tool delivers multiple alerts resulting in multiple tickets being created in the system of engagement.
At this point the analyst completes the root cause and works on that ticket, through remediation the other tickets get canceled, adversely wasting the analyst’s time. Due to the ticket cancellation, additional tickets stack up within the system of engagement.
In a more mature state of automation, AI drives event correlation, suppression and classification by identifying the parent alert. It then drives proper communication to service owners, users, and support groups.
Understanding Maturity and the Model
The output of machine learning runs on the input data such as IT elements, network elements, monitoring, and interdependency maps. These elements either live within or are integrated to a Service and Impact Aware CMDB, with additional enrichment coming from events, logs, and metrics. As the data begins to flow, we have the ability to apply three main techniques of learning:
- Unsupervised Learning: Clustering and dimension reduction
- Supervised Learning: Classification
- Reinforcement Learning: Optimized self-learning
To better understand where to apply each technique, lets look into the use cases:
Unsupervised Learning is generally more simplistic in nature, where we examine a set of given observations to identify patterns we didn’t know existed previously. Examples of these data sets are:
- Correlation between events and issues
- Reduction of alert noise
- Improved alerts pertaining to security anomalies
Supervised Learning, conversely, is the learn by example technique where the system needs to be told what is good or bad. An example of Supervised Learning in the field is SPAM emails, this is a good email, and this is SPAM. In this technique the system would be given multiple images of different letters and would be told what letter that image represents. As more and more examples are provided, it “learns” how to distinguish SPAM from other emails.
Reinforcement Learning, this technique focuses on rewarding desired behaviors and/or punishing undesired ones. This technique is based on trial and error to help the agent perceive and interpret its environment.
Benefiting from AIOps
As user confidence rises with AIOps outputs, so does adoption into everyday tasks and activities. This is where we start to realize scale and the emergence of the ability to drive innovation and cultivation of strategy. It is at this point the AIOps investment yields effective rate of ROI.
So where do we look to begin achieving this adoption and the use case opportunities? As an Elite Consulting partner on the ServiceNow platform, we shortcut this by running what is known as Automation Discovery in key areas leveraging your data. Along with this we seek to employ preferred tag standards and assurance of observability:
- Performance Monitoring: We proactively seek opportunities to remediate and optimize performance issues in real time.
- Infrastructure Topology: Our focus in on making static maps actionable and generating near real time visibility. This coupled with being able to look back at historical versions, allowing you to be able to answer what happened and what is happening?
- Noise Reduction: We proactively look at use cases to drive away from alert fatigue and move towards a model that supports filtering and correlation of meaningful data delivering intelligent alerting.
- Anomaly Detection: Organizations struggle in root cause/problem management, making anomaly detection a critical discipline. In AIOps, we focus on the difference between the value of a KPI and what the machine learning model predicts. This allows us to flag deviations and focus attention effectively.
Having the proper AIOps methodology is critical in the success of the program. What stands in the way of success are consistent challenges:
- Expertise: this is one area you cannot develop your way through, it requires data and process expertise
- Infrastructure: this requires a federated model to a system of engagement with multiple, use case based, data sources
- Time to Value: without a specialized and proven methodology, AIOps TTV can be slower than what you anticipated
- Data: volume, quality, and consistency of data produced can get overwhelming and requires skilled modeling
These challenges only represent your risk and cannot be a deterrent from progressing. We can effectively push through them by recognizing and addressing them and ensuring we are focused on the proper use cases. By instituting a best practice use case identification model we can quickly find the common use cases in your environment. For example:
- Predicting incident assignment
- Incident classification using natural language
- Finding similar incidents to accelerate resolution
- Name entity recognition for faster processing
- Grouping or clustering of alerts based on symptoms or description
- Correlating events
- Forecasting value of metrics to prevent outage
- Problem identification based on anomalies
Final Word
In closing, adopting and strategically planning an AIOps strategy sounds cumbersome and can be. Understanding that it can be broken out into two segments, automated service and automated operations is important.
Automated Service: enhances value co-creation by facilitating outcomes that the customer wants to achieve through automated task fulfillment
Automated Operations: leverages machine learning and automation to minimize work efforts associated with day-to-day activities, processes, and infrastructure that are responsible for delivering value to the business through technology
A great starting point for most organizations that use ServiceNow as their system of engagement is immediately focusing on, automated service. This is because the only challenger element standing in your way is quality and volume of data. To launch this, the focus needs to be on adoption of the platform at the user and service layers. What makes this easier to launch is our ability to start at the incident or request tables to identify your unique automation candidates vs having to create the entire federated operations model. This requires fewer Centers of Excellences (COEs) to be involved and focuses more on a critical impact area, user experience! By doing this we are able to prove out the automation capabilities and provide factual value stories that empower executives to seek further funding for AIOps expansion and generates an actionable roadmap for execution.
Subscribe to Our Blog
Related Posts
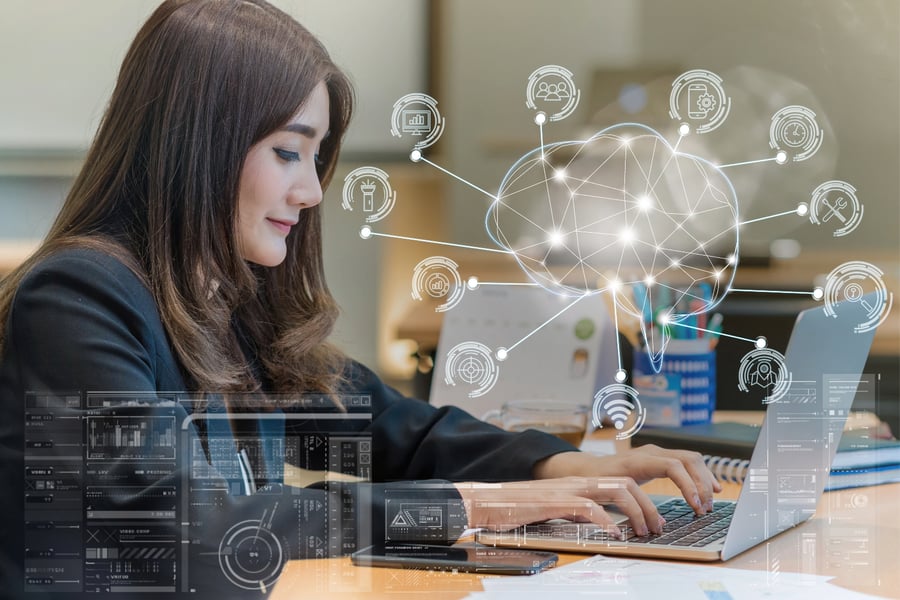
Simplifying the Delivery of AI: Best Practices for Effective Implementation
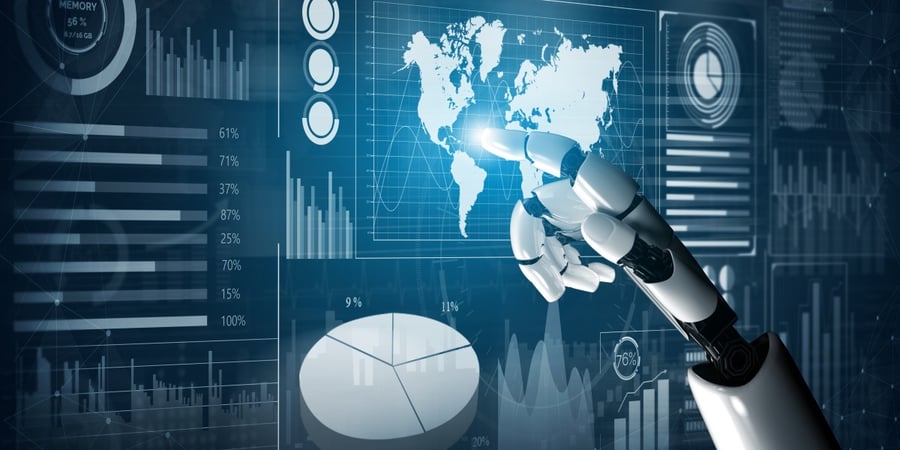